The role of a Property and Casualty insurance adjuster is challenging. This multifaceted role involves overseeing contractors, experts, accountants, and legal counsel while simultaneously addressing the emotional needs of the insured.
Traditional adjuster support systems, like experienced managers, are often constrained by time limitations and may not be consistently accessible. While training programs aim to equip adjusters with essential knowledge, the diversity of claim scenarios makes it difficult to cover every situation comprehensively. As a result, adjusters often face the challenge of making important decisions based on their own best judgment, without a reliable sounding board. This lack of a centralized decision making can result in inconsistent decisions and payouts across different adjusters, a phenomenon commonly known as decision noise.
In his book “Noise,” psychologist and Nobel Laureate Daniel Kahneman defined decision noise as the undesired variability in professional judgments. Kahneman has studied noise for decades and provides a glimpse of noise within insurance organizations. After surveying over 800 insurance CEOs, Kahneman discovered that insurance executives believed there was noise in their organization, estimating the level of noise to be approximately 10% (one insured might receive a payout of $9,500, while another could receive $10,500 for the same loss). However, subsequent audits conducted by Kahneman revealed a substantially higher noise level of 43%, a large deviation from the ideal level of consistency expected within a highly regulated insurance organization.
Noise may appear to be a problem that ‘balances out’, some adjusters underpay while others overpay. However, noise has adverse consequences. Underpaid insureds are likely to be dissatisfied and more likely to switch insurers, making it challenging to recover payouts. Overpaid insureds contribute negatively to the loss ratio, affecting the overall profitability of the insurer.
Despite being a longstanding issue within the insurance industry, noise in decision-making has proven resistant to traditional methods of training and manual oversight, providing insurers with the incentive to explore AI, specifically Multimodal Large Language Models (MLLMs), as possible solutions to reduce decision noise.
Unlike conventional text-only models like ChatGPT, MLLMs process information by seamlessly integrating various modalities, including text, images, and audio. This unique capability enables MLLMs to simultaneously delve into claim notes, analyze claim photos, and interpret phone conversations, developing a nuanced understanding of a specific claim.
In our collaboration with several leading insurers, we observe a keen interest in deploying AI models as intelligent claim companions. MLLMs have the potential to become well-versed in the intricacies of a claim, and offer real-time input and assistance to adjusters during decision-making. The companions could theoretically play a vital role in helping the adjuster interpret a photo from the insured early in the claim, where uncertainty is at its peak.
An illustrative example of such a model is shown in the photo below. The screenshot was generated by Mitigateway during our testing using an open-source MLLM called Llava, running without internet access (locally). It demonstrated the local model’s ability to create relevant follow-up questions from a single photo and a rather simple, zero-shot, vague request.
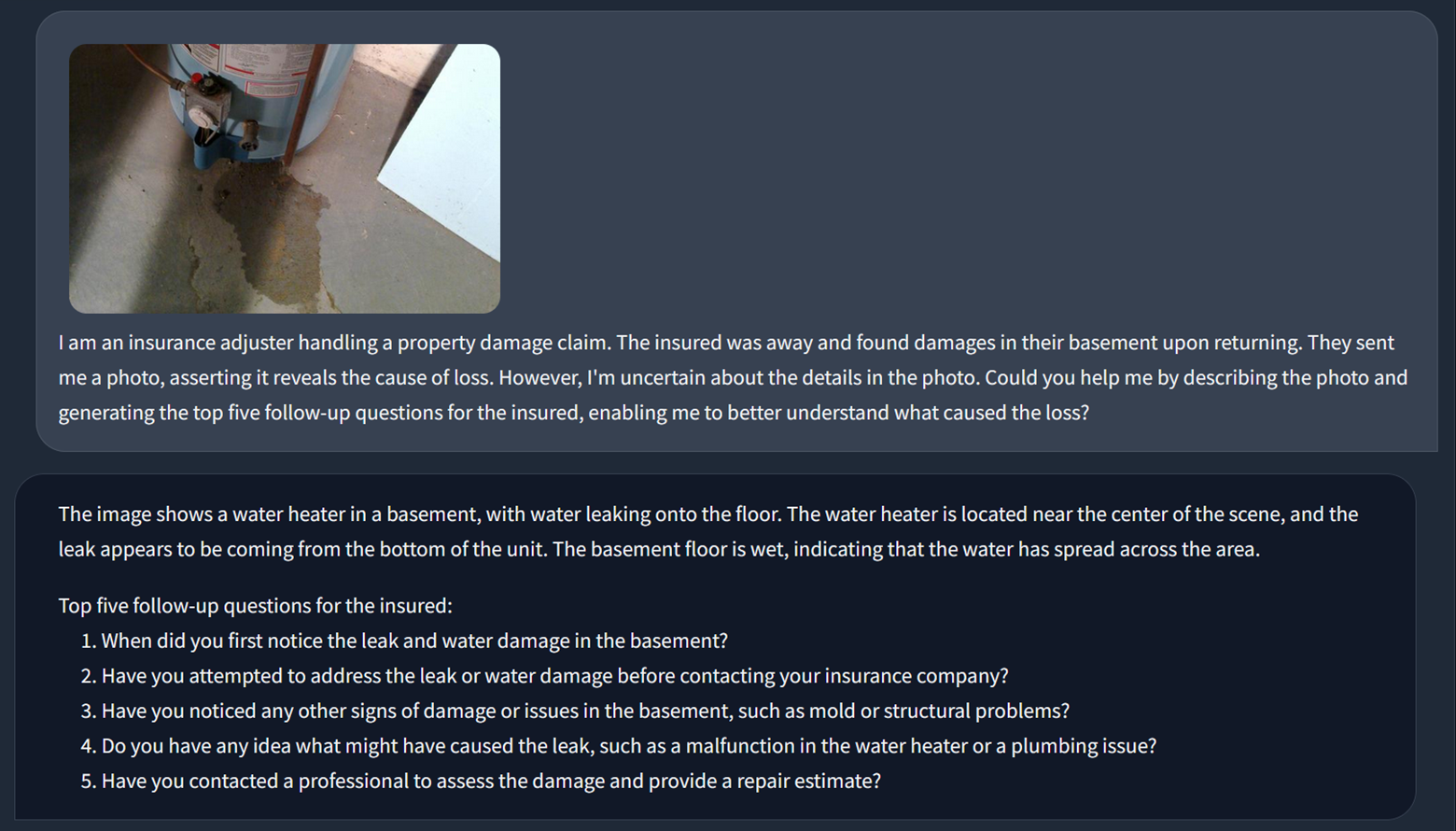
Once integrated, these AI claim companions could aid adjusters with decision making, minimizing decision noise. Additionally, these companions have the potential to perform many clerical tasks and preserve the cognitive resources of adjusters for more important tasks. MLLMs have the potential to transform adjusters into genuine advisors for the insured, offering personalized guidance and significantly elevating overall customer satisfaction.
The concept of using AI tools to minimize insurance decision noise is promising. However, our insight indicates that the current models and processes for integrating AI as claim companions are not fully developed. Consequently, some insurers perceive allocating resources to experiment with these ideas as too risky, particularly given the uncertain timeline for when these tools will generate a positive ROI.
To de-risk these initiatives, we recommend that insurers identify small, niche projects for deploying AI models locally. Having experience with these tools ourselves, we see many positive ROI projects for deploying AI tools, and we are working with insurers who want to solve smaller scope projects with these tools, for example, in identifying subrogation, claim leakage, or simply creating structured data (sortable and searchable) from unstructured data, such as long-form text and images.
Existing AI models and processes could provide insurers with insights into their extensive repository of claim history, offering valuable information on risks and causes of claim leakage.
Deploying these small-scale initiatives would create positive ROI projects that enable insurers to build out AI testing and governance frameworks, design processes for how claim data could be processed by AI, and test the capabilities of existing models on their own data. Such projects would develop internal expertise in operating these models and keep the insurer up to date on emerging model trends.
Considering the frequent release of new and more powerful models (before 2023, there were no powerful open-source models, and now there are many), only insurers who are ready to experiment, have the existing frameworks, and are familiar with these innovations will be able to maintain a competitive edge.